How reliable are input reads for spike-in-free ChIP normalization?
2022 May 25
ChIP
Normalization
R
Question 1: how much do total input reads agree with the actual sample size?
Question 2: does any input sub-region disperse from the sample size?
Question 3: do ChIP reads correspond to the total input reads (sample size)?
- Sub-question 3-1: do ChIP background reads correspond to the total input reads (or sample size)?
- Sub-question 3-2: do ChIP signals on the invariable/non-specific genes represent the sample size?
Before answering these questions, spike-in-free ChIP normalization has a collection of existing methods esstimating sample size with different source of read count (RC):
Method | Description | Reference |
---|---|---|
Total input | Scale ChIP with input total RC | Kumar et al. 2019 |
Total ChIP | Self-scale with ChIP total RC | Marks et al. 2012 |
Background | Minimum ChIP/input ratio in bg bins | Liang et al. 2011 |
Background | Sorted bg bins max cumsums.diff of ChIP-control | Diaz et al. 2012 |
Peak | Tipping point of ChIP density | Jin et al. 2020 |
Peak | Anchor genes as ChIP size reference | Polit et al. 2021 |
It’s easy to find among the methods, two major sources of variation are aimed to adjust:
- different amount of starting material—assuming input as sample size
- different efficiency of pull-down—assuming bg as invariable contamination
The input normalization has achieved ideal scaling results in pre-mixed H3K27me3 dataset, although small fluctuation still exists (Kumar et al. 2019). This dataset also leaves a open question to the background normalzation idea, the question 3-1. Since the dramatic changes of ChIP background reads after H3K27me3 increase/decrease by GSK/Ezh2i also suggests the dominant “ChIPability” over the non-specific contamination.
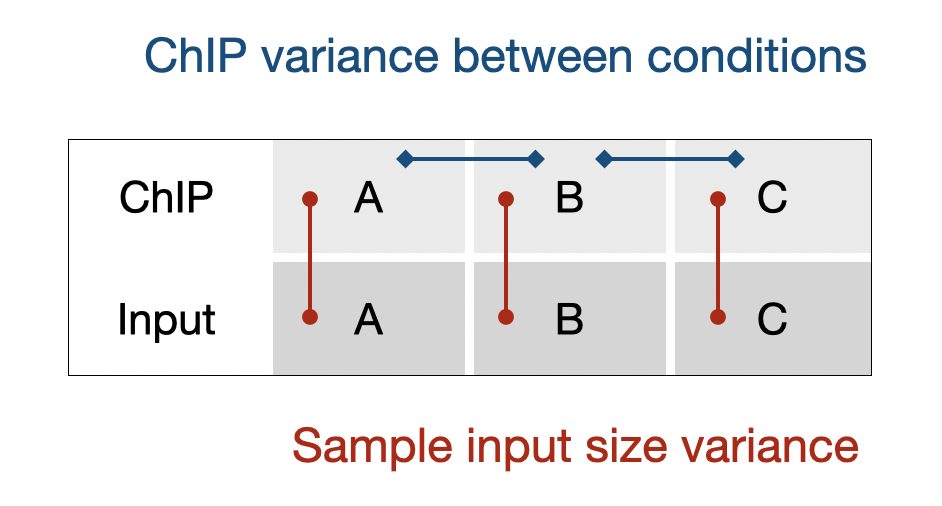
Vertical (input) and horizontal (ChIP) normalization
But input (vertical) and background (horizontal) normalization are not totally incompatible. When ChIP targets global change (in certain regions) are expected to be minimal, the main sources of input-normed ChIP variation will be subjected to the input RC variance (PCR, barcode, pipetting error, etc.), and ChIP variance (PCR, barcode, ChIP quality, etc.).
So an additional background-norm can address both variances. However, if the ChIP target has dramatic changes between conditions (>10 folds), the technical variance will become unnoticeable, and the horizontal variance will largely exhibit the biological effects.
So the reliability question is subjected to the type of ChIP — the smaller the change, the larger fraction of technical errors, therefore the less reliable of using only input RC size. For example, a stable target in 3 ChIP samples (A, B, C) could appear 15% changes between conditions which is from purely technical issues; however, if a target changed dramatically, 10-fold or 1000%, the ground truth will override the small technical variance. In the first case, the input RC global normalization would inevitably introduce false positive p-values at the genome-wide level, but insignificant for the second case.
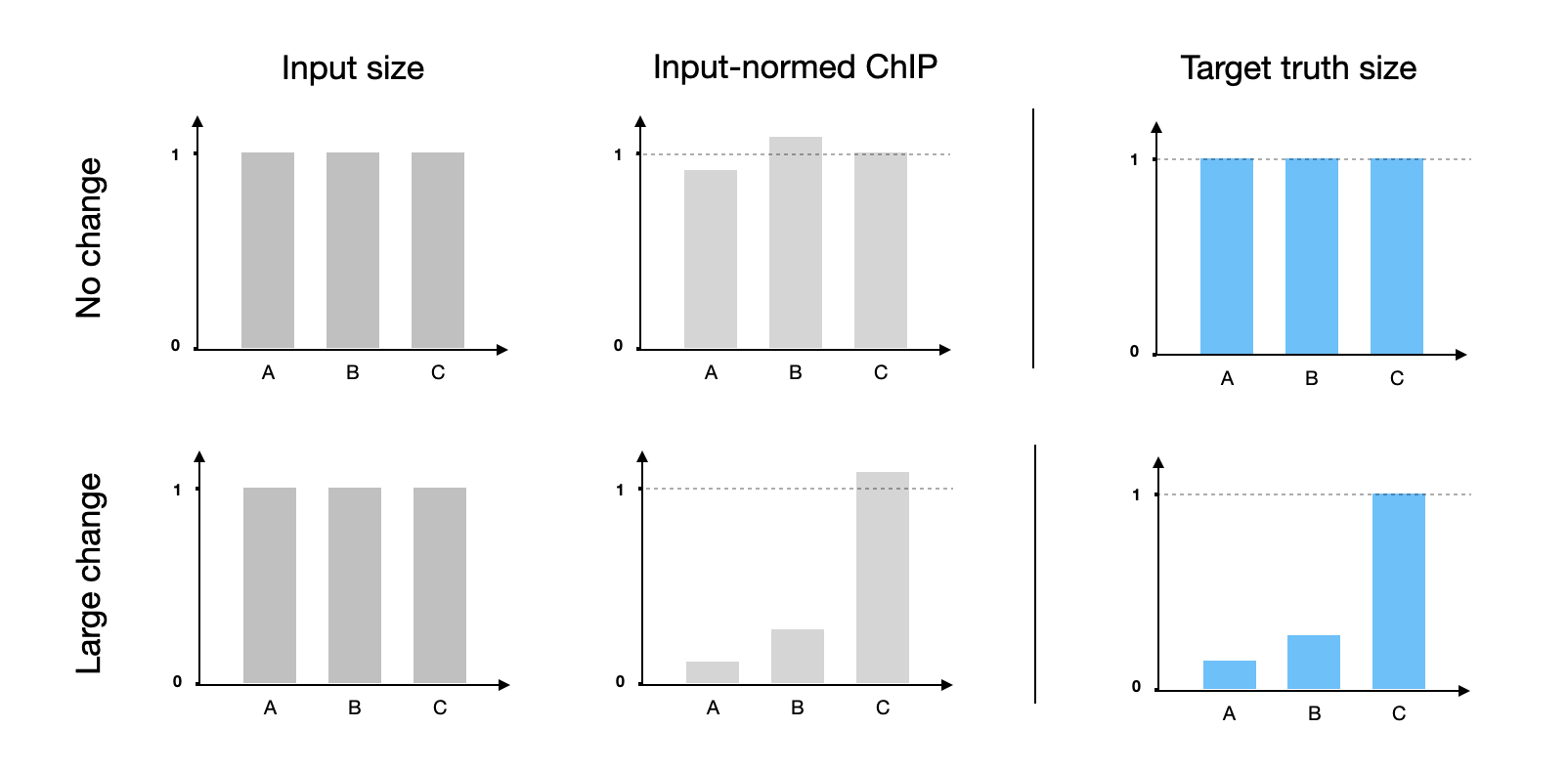
Degree of real changes influence the input normalized ChIP variance
The conceptual issues has been discussed so far. The pratical issue how to migrate from pair-comparison to multi-comparison of actual sequencing runs will be helpful with the beginning questions in coverage.